Prediction of freshwater harmful algal blooms in western Lake Erie using artificial neural network modeling techniques
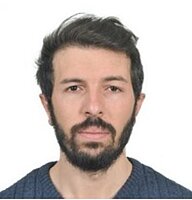
Haci Osman Guzel is a Master of Science student in the Natural Resource Management program at North Dakota State University. He completed his bachelor’s degree in Agricultural Engineering at Adnan Menderes University, Turkey. His research area is to apply mathematical modeling techniques to simulate harmful algal bloom distributions in freshwater environment.
Email: haciosman.guzel@ndsu.edu
Fellow: Haci Osman Guzel
Advisor: Halis Simsek, Ph.D., Assistant Professor, Agricultural & Biosystems Engineering Department, North Dakota State University.
Degree Progress: M.S. in Natural Resource Management expected graduation Fall 2018.
Prediction of freshwater harmful algal blooms in western Lake Erie using artificial neural network modeling techniques
Excess nutrients in a freshwater environment stimulate blue-green algae, which rapidly increase and accumulate in lakes and rivers when the optimum environmental conditions are met. Cyanobacteria are a type of blue-green algae that form harmful algal blooms (HAMs) in water ecosystems. They are prokaryotic organisms called CyanoHAB. The Cyanobacteria genera includes Microcystis spp., Planktothrix spp, Anabeana spp. Cylindrospermopsis spp., Aphanizomenon spp., and Oscillatoria spp. and are the major environmental concerns in freshwaters production of toxin (referred to as a cause of animal and human poisonings or health risk) and cyanobacterial metabolites in aquatic systems.
Development of a HAB early-warning system is highly dependent on the reliable modeling methods that predicts the HAB occurrence with a high accuracy. In order to minimize the impact of HAB in aquatic system, the current situation and upcoming forecast should be evaluated using an appropriate model. Based on the available data, the type of model can be selected to estimate HABs occurrence in freshwaters. Artificial intelligence techniques, in particular artificial neural network (ANN) techniques have been used extensively in a variety of complex scientific and engineering problems to predict and classify environmental systems including system modeling, forecasting, hydrology, pattern recognition, sediment transport and accumulation, evaporation, evapotranspiration, rainfall, surface runoff, and watershed runoff. However, the application of these techniques to HABs estimation is very limited in the literature. For modeling purposes, the essential parameters to be considered in the rivers and streams that effect the occurrence of HABs are as follows; nutrient loading, water temperature, volumetric flow rate, water current and turbulence, residence times of water, sunlight exposure time and intensity, quiescent or stagnant water, and depth of the water (deep or shallow). Some or all of those parameters might be used to develop the ANN models.
Project Objectives:
The main objective of this study is to develop and apply ANN modeling to predict HABs in wester Lake Erie (Ontario, Ohio). The specific objectives are as follows:
- To determine contaminated freshwaters with cyanobacteria in the USA and obtain the necessary parameters to run the model
- To determine the best estimation input parameters for a selected freshwater
Progress:
The entire western end of Lake Erie, designated by Ohio as an impaired waterway, was selected due to serious contamination problems with cyanobacteria, and most importantly, a number of data were available for this lake to evaluate the lake’s contamination level. Total eight input parameters including phosphorous, nitrogen, chlorophyll-a, air temperature, water temperature, turbidity, wind speed, and pH were determined to estimate blue-green algae. These input parameters were determined based on the lake’s environmental conditions and the data availabilities. National Oceanic and Atmospheric Administration (NOAA) Great Lakes Environmental Research Laboratory (GLERL) (NOAA-GLERL, 2018) obtained the real-time buoy data from the lake. The data were collected by NOAA-GLERL in the years of 2016 and 2017 and saved using PostgreSQL database management system, which was a powerful, open source object-relational database system.
Significance:
Cyanobacterial toxins (cyanotoxins) cause lethal effects on living aquatic organisms. The overabundance of HABs in freshwater increases the risk to human and animal health, reduces water transparency, creates oxygen-deprived aquatic zones, leads to death of dwelling plants and fish, effects biodiversity, and ultimately decreases the recreational use of water. Nutrient over-enrichment in a freshwater ecosystem is mainly linked to human activities, including agricultural uses of fertilizer, application of manure, discharge of municipal wastewaters, and inputs from industries. HAB is especially important if the water is used as a municipal drinking water reservoir.
As of our knowledge, this is the first study to estimate HABs using ANN techniques. Advances in environmental monitoring techniques and generating extensive and continuous water quality parameters promote using these techniques to estimate HABs in freshwaters. This research will provide important outcomes to improve the quality of freshwaters by minimizing the nutrient entrance to the freshwaters from point or non-point sources. Additionally, the models that obtained in this study could be valuable for regulatory agencies when evaluating the future contamination of freshwaters.
Research Outcomes:
Haci Osman Guzel, Bilal Cemek, Dean D. Steele, Halis Simsek, 2018. Prediction of freshwater harmful algal blooms in Western Lake Erie using artificial neural network modeling techniques. Presented at ICSAE 2018 5th International Conference on Sustainable Agriculture and Environment, Oct. 8-10, Hammamet, Tunisia.
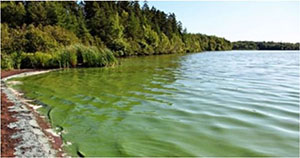
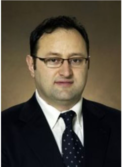
Halis Simsek
AES Ag & Biosystems Eng
Office: ABEN 2016
Phone: 701-231-6107
Email: halis.simsek@ndsu.edu