☼ Spotlight on Faculty Research ☼ |
An empirical investigation of buyer–supplier relationship typologies and their behavioral and performance outcomes
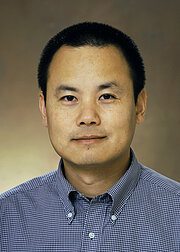
Jin Li, PhD
Professor of Marketing
Business buyers and suppliers can form many different types of relationships. The existing literature proposes four classical and four emerging buyer–supplier relationship (BSRs). In this study, Dr. Jin Li collaborated with Drs. Linlin Chai, Chanchai Tangpong, Michelle Hong, and Rodney D. Traub to conduct an online survey, collecting data from 371 purchasing managers in the USA. They aimed to empirically investigate BSR typologies and their behavioral and performance outcomes. Their statistical analysis supported the existence of five out of these eight BSR types, including strategic/bilateral partnership, market/discrete, supplier-led collaboration, captive supplier/buyer dominant, and captive buyer/supplier dominant BSRs. Furthermore, they found that these five BSRs differ in terms of behavioral outcomes and performance measures.
Managers should consider the potential to develop and enhance a strategic/bilateral relationship with their supply chain partners, which, in at least some circumstances, can lead to superior performance results. Similar observations can be made with respect to supplier-led and, to a lesser degree, buyer-led collaboration.
https://doi.org/10.1108/JBIM-06-2020-0281
Reporting misstatements as revisions: An evaluation of managers’ use of materiality discretion
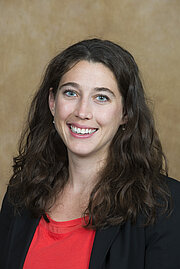
Rachel Thompson, PhD
Assistant Professor of Accounting
In recent years, firms reporting revisions of prior financial statements outnumber those reporting restatements. Misstatements that are material to prior periods are required to be reported as restatements, whereas immaterial errors can be reported as revisions. Based on SEC guidance and widely used materiality benchmarks, I find a significant percentage, 29%, of revisions are suspect in that they meet at least one materiality criterion. These suspect revisions are 15% to 29% more likely to be reported when managers have a strong incentive to avoid restatements—when they face the threat of a compensation clawback for reporting a restatement. This result is especially salient when the clawback policy does not require misconduct for recoupment and when the error correction significantly reduces prior period net income. Overall, this evidence suggests that some managers use materiality discretion opportunistically to report misstatements as revisions instead of restatements.
https://onlinelibrary.wiley.com/doi/abs/10.1111/1911-3846.12877
Defined Benefit (DB) Pension De-risking Project
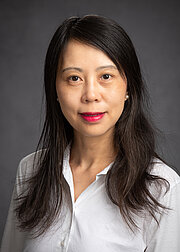
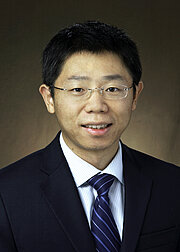
Ruilin Tian, PhD
Professor of Finance
Jeffrey (Jun) Chen, PhD
Associate Professor of Finance
Defined Benefit (DB) pension plans are confronted with macroeconomic swings, volatile funding levels, increased longevity, and new regulatory requirements, necessitating effective risk management strategies. With the support of the Society of Actuary (SOA), Dr. Ruilin Tian and Dr. Jeffrey (Jun) Chen spearheaded an innovative research collaboration exploring the intricate landscape of DB pension plans and their risk transfer strategies (i.e., pension de-risking), with a focus on the U.S. context over the past three decades.
The project navigates challenges posed by limited publicly available data on U.S. companies' pension de-risking activities. Collaborating with Dr. Limin Zhang, we conducted exhaustive web crawling of 30 million filings in the SEC Edgar database, employing text mining, machine learning, and manual validation to construct a unique pension de-risking database that encompasses a whole spectrum of distinctive risk transfer activities.
The outcomes culminate in multiple papers and technical report, which explored the determinants of pension de-risking, dissected the dynamics of pension assets and de-risking-adjusted liabilities, analyzed the interplay between business environment and pension de-risking decisions, and evaluated the economic impacts of pension de-risking. The related pension research can be found in the SOA report, “De-risking Strategies of Defined Benefit Plans: Empirical Evidence from the United States”, and “Applications of Machine Learning in Text Identification for DB Pension Analysis” published at Risks. More working papers targeting Insurance: Mathematics and Economics, Journal of Risk and Insurance, andJournal of Corporate Finance are under development.
The study bridges a significant gap in the pension risk management literature by furnishing empirical evidence from the U.S. market. With relevance to academics and practitioners alike, the findings extend beyond borders, offering valuable insights to corporations, pension plan sponsors and trustees, investors, and regulators for informed de-risking decisions.
- Ruilin Tian and Jeffrey (Jun) Chen, De-risking Strategies of Defined Benefit Plans: Empirical Evidence from the United States, Society of Actuarial (SOA), 2020.
https://www.soa.org/resources/research-reports/2020/de-risking-strategies/
- Limin Zhang, Ruilin Tian, and Jeffrey (Jun) Chen, Applications of Machine Learning in Text Identification for DB Pension Analysis, Risks, Volume 10, Issue 2, 41, 2022.
DOI: https://doi.org/10.3390/risks10020041